We are happy to announce that our paper entitled “Invisible but Detected: Physical Adversarial Shadow Attack and Defense on LiDAR Object Detection” has recently been accepted for publication in the Proceedings of the 34th USENIX Conference on Security Symposium (USENIX Security 2025). Congratulations, Kobayashi-kun and the team!
Ryunosuke Kobayashi, Kazuki Nomoto, Yuna Tanaka, Go Tsuruoka, Tatsuya Mori, “Invisible but Detected: Physical Adversarial Shadow Attack and Defense on LiDAR Object Detection,” Proceedings of the 34th USENIX Conference on Security Symposium (USENIX Security 2025), August 2025 (to appear).
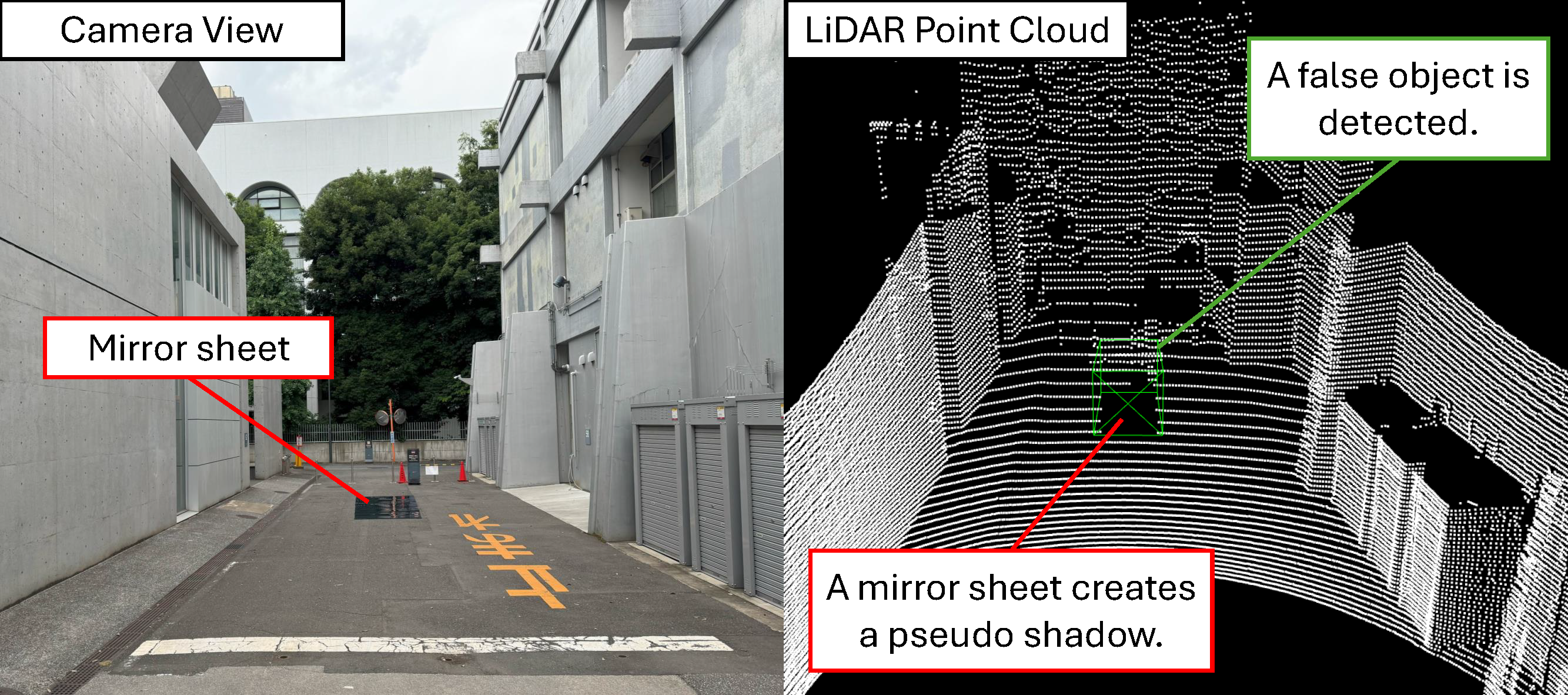
Overview.
This study introduces “Shadow Hack,” the first adversarial attack leveraging naturally occurring object shadows in LiDAR point clouds to deceive object detection models in autonomous vehicles. Unlike traditional adversarial attacks that modify physical objects directly, Shadow Hack manipulates the way LiDAR perceives shadows, affecting detection results without altering the objects themselves.
The key technique involves creating “Adversarial Shadows” using materials that LiDAR struggles to measure accurately. By optimizing the position and size of these shadows, the attack maximizes misclassification in point cloud-based object recognition models. Experimental simulations demonstrate that Shadow Hack achieves a 100% attack success rate at distances between 11m and 21m across multiple models.
Physical-world experiments validate these findings, showing a near 100% success rate at 10m against PointPillars and 98% against SECOND-IoU, using mirror sheets that remove almost all LiDAR-detected points from 1m to 14m. To counter this attack, the authors propose “BB-Validator,” a defense mechanism that successfully neutralizes the attack while maintaining high object detection accuracy.
This work highlights a novel and critical vulnerability in LiDAR-based perception systems and presents an effective defense, contributing to the ongoing effort to enhance the security of autonomous vehicles.