We’re excited to announce the arrival of four new PhD students in our lab, bringing our total number of PhD students to an impressive ten! Three of these new PhD students are pursuing their studies through a unique professional doctoral program, where they continue their work at their respective companies while advancing their research with us. This blend of academic and professional experience enriches the diversity and depth of knowledge in our lab.
Our master’s students also continues to grow, with thirteen students currently pursuing various advanced studies. In addition, we are pleased to welcome eight new undergraduate students. These students are the future of our research efforts, and their energy and fresh perspectives are invaluable to our team.
The range of research topics in our lab is as diverse as it is fascinating. Our work ranges from AI security and autonomous vehicle security to Web3 security, human factor security, physical space authentication techniques, and cybersecurity. This breadth of study reflects our commitment to pushing the boundaries of what’s possible and addressing some of the most pressing challenges in technology and society today.
We’re excited to see the contributions these new and continuing students will make to their respective fields. Their hard work and dedication make our lab a vibrant and innovative place. Welcome aboard, everyone! Let’s make great strides in research together!
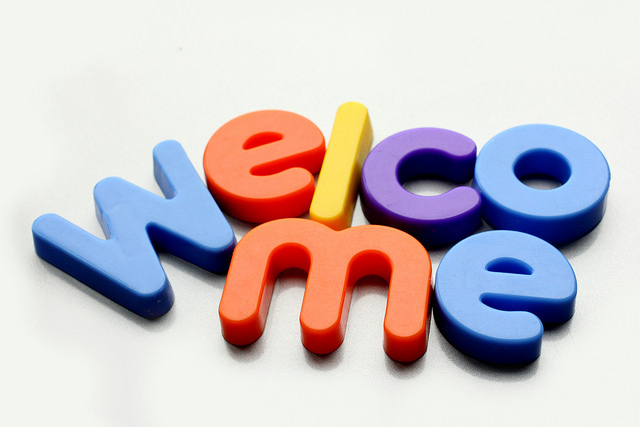